Spatial and visual comparison analysis of health disparities in London neighbourhoods: the case of Southwark and Lambeth
Highlight box
Key findings
• Spatial disparities in health outcomes can differ within two contiguous boroughs due to varying socio-economic and environmental factors.
• Poorer health outcomes are associated with overcrowding and deprivation.
• Better health relates to a better provision of public green and sports facilities and low levels of population, building density, and deprivation.
What is known and what is new?
• Different methodological approach focused on granular approach to the selection of geographical areas to study. Comparing side-by-side neighbourhoods characterised by high demographic and urban form differences.
• Confirming that health and socio-economic factors are associated.
• Considering the role of private green spaces, community services, sports and play facilities in promoting health and well-being.
What is the implication, and what should change now?
• Affirming the significance of conducting a more comprehensive analysis and employing a wider variety of metrics in understanding health inequalities. This encompasses an array of spatial metrics, particularly those related to the built environment.
• There is a need to target initiatives that promote better access to green spaces, recreational areas, sports facilities, and community spaces.
Introduction
In the aftermath of the coronavirus disease 2019 (COVID-19) pandemic, there is a growing discourse concerning the impact of urban planning and city living on health and well-being (1,2). As cities and local neighbourhoods return to a semblance of normalcy, there has been a ‘renewed’ sense of urgency among policymakers and health managers regarding how the way we plan and live in cities can affect our health and well-being (3,4). While it is widely acknowledged that socio-economic and spatial conditions play a significant role in various physical and mental health outcomes, the analysis of how spatial variations at the borough and neighbourhood level compare with divergent health levels, while gaining recognition, remains relatively unexplored (5-7).
To address this gap, this paper takes a localized approach to examine spatial patterns of health, often referred as urban health inequalities (8). It does so by mapping patterns of socio-economic and environmental factors across different neighbourhoods and charting the relation between these factors and health, within 12 carefully selected neighbourhoods in London. Using data visualisation methods supported by Geographic Information System (GIS) analysis and mapping, this study creates a comparative spatial compendium to enhance the understanding of health disparities among residents of Southwark and Lambeth, two central London boroughs chosen for their diverse urban environments and varying health levels within their populations.
Importantly, this study does not aim to establish direct causation between urban determinants and health outcomes. Instead, it aims to add a spatial dimension to health profiles, enhancing the understanding of the intricate relationship between location and residents’ health. The goal of this research is to shed light on the complex dynamics between urban environments and health, foster the development of a place-based approach to addressing urban health challenges, and provide valuable insights for future planning and policy considerations and highlight factors and outputs that might be explored further using other methodologies. The relevance of this paper is an attempt to start a discussion about some of the outputs of the analysis and to influence policy making and management.
Literature review
Considerable research effort has been devoted in recent years to describing, and seeking to understand, place-to-place variations that portray health inequalities at a variety of spatial scales (9-14). At the same time, public health practitioners also recognise the need for collaborative efforts beyond traditional health programs to address policy decisions impacting environmental quality and health (14) and where “environmental health reunites with architecture and urban planning” (13).
Within this analysis, the key issue is the exploration of patterns that might relate health variations, the socio-economic characteristics of residents, and the characteristics of the areas in which people live. In order to tease out the relative importance of these factors, multi-level quantitative modelling has been the preferred mode of analysis while the physical geographical space has been the space or ‘backcloth’ onto which health inequalities have been projected (15,16). While the relation of socio-economic factors and health has been better explored in the literature, the role of the physical environment in contributing to the enhancement of that same relation is still within a long path until its full maturity (17,18).
If COVID has replicated some health inequalities, it also alerted policy makers to the role of space in addressing these inequalities (19). The role of the neighbourhoods as a unit of analysis, has also shown to be an important context for health promotion (20,21) going beyond the socio-economic composition of the population, to the exploration of their physical environments and their complex interactions, which determine health behaviours and outcomes. Evidence suggests that individuals who live in places with underprivileged social and physical environments are at higher risk of different health conditions and mortality (18,20-22). However, there are many missing links in the current exposure assessment (17), and in the translation into place-based interventions, backed up by social sciences and spatial analysis.
The choice of a neighbourhood or ‘place’ as a unit of analysis often emerges in the literature as a proxy for understanding the interactions between individuals, lifestyle choices, and the environment (23-25). Understanding the mechanisms through which places influence health can aid in identifying health issues concentrated in specific areas, which is essential for the effective allocation of resources for health issue prevention. Moreover, recent advancements in geographical information systems and the increasing availability of public health databases with recorded locations have provided numerous researchers and public health practitioners with the tools to analyse spatial data (23).
Most research on place effects has made serious efforts to determine the extent to which places genuinely impact health variations. This was achieved by trying to distinguishing between (I) contextual effects—the characteristics (social, material, environmental, or political) of the areas in which people reside; and (II) compositional effects—the social characteristics and material circumstances of the individuals living in those areas (9,22,24,26,27). Some studies have explored this relation within quantitative approaches (21), or narrowing down to behaviours, as walkability (6), which often misses out on the comprehensiveness of the spatial characteristics of these neighbourhoods. However, much less attention has been given to investigating the mechanisms and pathways through which people’s health might be affected by the complexity of their residential context (22). This complexity includes factors such as (I) features of the environment equally shared by all residents in a locality (including air pollution, for example); (II) availability of healthy home, work, and leisure environments (including green spaces or healthy foods, for example); (III) services and facilities to support daily living (including transport networks or sports and cultural facilities); (IV) sociocultural features (including the community’s history, social norms or social capital, for example); (V) neighbourhood reputation (as stigmas, and reflections on morale) (26). However, there are obvious interactions between these categories, and they are not mutually exclusive. Health variations also depend on contextual factors, and a more comprehensive strategic planning with an impact on residential, social, and physical environments is essential (22,25). This study aims to explore the influence of the complexity of people’s residential environments on their health. It examines the mechanisms and pathways involved and uses spatial pattern analysis as the approach and explores known determinants of health that capture some of the complexity described by Macintyre, Maciver, and Sooman (26), such as levels of housing density, public transport access, walkability, deprivation, and crime that interact with neighbourhood-level demographic characteristics in complex ways (20).
However, it is important to note that comparing health levels between different residential neighbourhoods may be confounded by the omission of variables which could also impact on the results, leading to non-exchangeability across neighbourhoods (17). Another limitation of these studies is the sole focus on residential neighbourhoods, which can introduce substantial uncertainty in research results, because people spend a considerable amount of time outside their home environment (22,28), or when the administrative and/or statistical areal units do not correspond to people’s true geographic contexts (22).
Nevertheless, even considering these limitations, this examination can suggest spatial associations to explore in future research that would help clarify targets for policy intervention. Rather than developing hypotheses, the research aims to draw attention to health inequalities and stimulate policy discussions as investigations on place effects should guide public health interventions. This study will use a place-based approach to uncover pathways and help determine how place-based policies and interventions should be defined (22). The methodological approach goes beyond the statistical value of these areas (15,25), and explores their contrasting urban geographies, through spatial analysis and urban scale. Mapping exercises and the exploration of the spatial dimension of health inequalities can provide a common geographical reference for a combination of indicators measuring contextual features which improve the comparability of studies and the understanding of the spatial dimension of health inequalities (29). While studies at the national level have been conducted (23,29), and others have employed various visual mapping methods and approaches (30), there has been fewer explorations of London neighbourhoods using GIS mapping to investigate the interplay between contextual socio-spatial conditions and varying health levels
In summary, this exercise uses comparative socio-economic and spatial indicators mapping to analyse neighbourhood patterns in relation to health outcomes while building a granular picture—a ‘spatial compendium’—of 12 London neighbourhoods. The goal is to contribute to academic and policy debates on this topic.
Methods
The research sets out to create a detailed profile of Southwark and Lambeth, two similar central London boroughs with internal contrasting socio-economic and environmental characteristics. To do that, the research analysed a combination of 12 neighbourhoods, 22 contextual factors, and three health-related indicators. Publication of this paper adheres to the LSE Research Ethics Policy and Procedures, and it only uses publicly available and anonymized data. The methodology and selection of spatial and health parameters is explained below.
Southwark and Lambeth are two central London boroughs with similar histories, location and north-south orientation, and population sizes (respectively 319,000 and 326,000 persons). With over 600,000 residents, the boroughs have a joint population larger than that of Manchester, though in half of the space. They cover nearly 60 square kilometres (km2) of London, an area half the size of Paris or the size of Manhattan. They are rich in history, with their northern neighbourhoods having been part of the capital’s early sprawl as the City of London expanded first into Southwark and later Lambeth. However, these two inner-city boroughs present significantly diverse intra-borough urban contexts with a variation of built-up densities, open and green areas as they extend southwards from the River Thames toward London’s outer boroughs of Bromley and Croydon (as seen in Figure 1). The presence of these characteristics, combined with their on-going investment in urban regeneration, offers an excellent opportunity to explore the relationship between urban conditions and health.
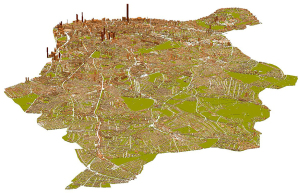
The historical backdrop of rapid growth, industrial change and migration has left the boroughs with a complex inheritance. Subsequently, both Lambeth and Southwark have become the home to immigrants from dozens of countries. The result is a complex tract of global citizens, with links both to Britain and the wider world who live and work in a highly fragmented, diverse, and dynamic urban environment at the centre one of the world’s major metropolises.
Both local authorities have relatively large concentrations of social housing, often alongside some of the most attractive (and expensive) homes in Britain, including Dulwich Park and Herne Hill. Some large housing estates proved challenging to maintain and have become home to some of London’s most deprived communities despite their central and well-connected location. As a result, areas like the Elephant and Castle have been substantially redeveloped in the past decade.
To ensure comparability of urban areas, and to better analyse and compare socio-spatial conditions within these boroughs, especially considering that not all data is disaggregated at the same level, this study used the UK government’s Middle Layer Super Output Area (MSOA) unit. The MSOA ranges from 5,000 to 15,000 residents as its statistical base and are used as neighbourhood proxies due to the readily available data.
To cluster and select a manageable sample of representative and diverse MSOA units within the boroughs, the research team used desktop research, GIS mapping, site visits, and local knowledge of the geographical, spatial, and social structures. The final selection aimed at representing the radically diverse urban contexts within the two inner-London boroughs. This included areas in the northern sections along the river (denser and more cosmopolitan), more mixed residential and commercial areas in the geographical centre, and some predominantly residential neighbourhoods towards the southern edges (see Figure 2). Along with their geography, a list of socio-spatial measures was used to help select objective urban characteristics of each area with a degree of precision. These were derived from various indicators employed by LSE Cities, on the Urban Age project and others, through a research methodology known as Urban Growth Analytics, serving as a framework for conducting international and national data-driven policy analysis of this nature (31,32). These included metrics such as: (I) index of multiple deprivation—where area scores where compared; (II) social housing—the comparison included the percentage of social rented housing; (III) population—where residential densities were used in the comparison; and (IV) ethnicity—where the highest non-white ethnic group was used in the comparison; and (V) differences in the urban form—where, for example, the green space coverage was used. Areas that have recently been redeveloped were excluded as available data would not reflect the recent changes to the social profile of residents.
This process was an attempt to select neighbourhoods that are bounded as MSOAs—corresponding to specific statistical unit of analysis—with similar geographic scale, similar number of residents, and some homogeneous or contrasting spatially based attributes (25,33,34). This paper will therefore designate MSOAs as neighbourhoods, even though the definition of neighbourhoods varies in the neighbourhood-health field (24).
The next step was to control for variation in health outcomes within the full sample of neighbourhoods. To do so, the selection and aggregation process considered three health indicators: female healthy life expectancy, childhood obesity (obese children in year 6) and diabetes prevalence. These were chosen for analysis as they can be influenced by socio-spatial factors, they include longer-term conditions or indicators with high variation within the case study areas and are of particular interest for the Impact of Urban Health organisation. A final clustering of neighbourhoods and health indicators was based in a process of matching neighbourhoods with positive and negative extremes of the three health indicators. The full process aimed at guaranteeing a rich sample and a more substantive analysis of the two boroughs.
Table 1 illustrates the final clustering process, where selected neighbourhoods were matched according to the distribution of diabetes prevalence, healthy female life expectancy, and obesity rates across the full sample. Using different symbols, extreme values within each category were highlighted, facilitating a more straightforward comparative analysis, to aid in the selection of areas.
Table 1
MSOA area name | Obese children in year 6, rank (value) |
Female healthy life expectancy at birth, rank (value) | Diabetes prevalence, rank (value) |
---|---|---|---|
Lambeth Walk & North Kennington | 10 (19.75)– | 6 (61.74) | 3 (6.27) |
Stockwell East | 2 (30.98)++ | 5 (62.35) | 5 (5.88) |
Stockwell West | 5 (27.04) | 10 (57.75) | 7 (5.65)– |
Loughborough Road | 1 (31.85) | 7 (60.34) | 2 (6.50)+ |
Tulse Hill | 6 (25.35) | 12 (56.84)– – | 6 (5.77) |
West Dulwich | 9 (23.13) | 4 (64.18) | 10 (4.78)– – |
Bermondsey North | 7 (25.00) | 2 (67.97)+ | 11 (4.77) |
Bermondsey East | 8 (23.55) | 8 (59.74)– | 8 (5.42) |
Burgess Park | 3 (28.65)+ | 9 (59.42) | 4 (6.19) |
Peckham North West | 4 (27.62) | 11 (57.18) | 1 (7.55)++ |
Peckham Rye Common | 11 (11.01)– – | 3 (64.32) | 9 (5.26) |
Herne Hill & Dulwich Park | 12 (9.33) | 1 (73.52)++ | 12 (4.21) |
Symbols used to denote best and worst outcomes range from (++) to (– –). MSOA, Middle-Layer Super Output Area.
To provide context for these neighbourhoods, which is essential for understanding the analysis and key outputs, the basic characteristics of each neighbourhood are described below.
Bermondsey East and Bermondsey North
In northern Southwark, between Tower Bridge and the Old Kent Road, this neighbourhood was initially industrial in the 18th century, thanks to its strategic location near the city and the port of London. Today, it’s a bustling area with a mix of upscale commercial and residential spaces, alongside Victorian terrace homes and social housing estates.
Peckham Rye Common, Peckham North West and Burgess Park
Burgess Park neighbourhood, located between Bermondsey and Peckham, boasts one of Southwark’s largest parks, replacing homes and factories destroyed during the Blitz. The area has a high density of social housing and high-rise residences, including the partially demolished Aylesbury estate. Peckham Rye Common features Victorian Peckham Rye Park and Peckham Rye Common, covering 0.5 km2 of green space. The neighbourhood is characterised by Edwardian and Victorian homes along long terraced streets. Peckham North West is a suburban residential area with various housing types, primarily housing low to middle-income households and social housing estates. It’s conveniently located within walking distance of the historic Rye Lane, a prominent shopping and architectural destination.
Herne Hill & Dulwich Park and West Dulwich
Herne Hill and Dulwich Park are vibrant areas known for their amenities, lively markets, and historic Herne Hill velodrome. While most 19th-century houses have been replaced by terraces, some remain in this ‘young professionals’ territory.
Tulse Hill
Tulse Hill, adjacent to Brockwell Park, is a diverse neighbourhood with a mix of semi-detached homes, terraces, and primarily rental council estates. It’s often described as a ’socially disadvantaged locality’.
Lambeth Walk & North Kennington
Lambeth Walk and Kennington, closer to central London, blend new developments with historic buildings and social housing. Despite ongoing gentrification, a diverse population of low to middle-income residents resides here, and the Oval Cricket Ground is a major attraction.
Stockwell East, Stockwell West, and Loughborough Road
Stockwell is a diverse neighbourhood with both middle-income and low-income residents. It features a mix of social and affordable housing units alongside Georgian and Victorian homes and large housing estates from different eras. While it has become less diverse due to gentrification, the population still maintains some diversity.
Following the selection and clustering of the case study areas, a list of predominantly environmental, but also social measures was developed. These measures and characteristics were used to define a representative sample of neighbourhoods in the two boroughs with a degree of precision. The final selection included 22 key parameters reflecting environmental (including physical) and socio-economic determinants selected to capture the urban character of each area (13,14,18). These include the nature of the building typology, the level of social and rented housing; the provision of street trees and green open space; accessibility by road, cycle, and public transport; car ownership and land use; distribution of cultural, educational and health facilities; levels of crime, overcrowding and deprivation; and other environmental factors including air and noise pollution. The selection of these indicators included the consideration of data availability and were limited to indicators that are available at that the MSOA unit of analysis. The full list of the selected indicators and their sources is presented in the table below (Table 2).
Table 2
Themes | Parameters: data source(s) |
---|---|
Urban health | |
Health outcomes | Female healthy life expectancy: provided by IoUH |
Childhood obesity: provided by IoUH | |
Diabetes prevalence: provided by IoUH | |
Boundaries | MSOA boundaries and total area (km): UK Data Service [2011] (https://borders.ukdataservice.ac.uk/easy_download_data.html?data=England_msoa_2011) and London Datastore—Statistical GIS Boundary Files for London [2011] (https://data.london.gov.uk/dataset/statistical-gis-boundary-files-london) |
Determinants of health | |
Environmental determinants | Buildings: number of buildings, built-up area, % of built-up area and % of open space—building footprint—OS MasterMap Building Attribute, Edina DIGIMAP Mastermap (October 2019) (https://digimap.edina.ac.uk) |
Average building height: relative height from ground level to base of the roof (RelH2)—same data source as mentioned above | |
Housing estates: estates net density where the comparison involved the combined footprint of all social housing buildings over the total of the area footprint. Social Housing Estates data was collected from Lambeth, Open Mapping Data [2020] (https://lambethopenmappingdata-lambethcouncil.opendata.arcgis.com/); and Southwark Maps [2018] (https://geo.southwark.gov.uk/connect/analyst/mobile/#/main) | |
Cycling infrastructure: paths and parking—TFL [2016–2021] (https://cycling.data.tfl.gov.uk/) | |
Public transport infrastructure: included bus routes and stops, road network and nodes—OS VectorMap District [2021] (https://osdatahub.os.uk/downloads/open); and rail network and stations—OS VectorMap District [2021] (https://osdatahub.os.uk/downloads/open) | |
Medical care facilities: GP practices and number of patients per GP—NHS Digital, Patients registered at a GP practice [2021] (https://digital.nhs.uk/data-and-information/data-tools-and-services/data-services/general-practice-data-hub) | |
Sports and play facilities: number and area per resident—OS MasterMap Greenspace, Edina DIGIMAP Mastermap (October 2020) (https://digimap.edina.ac.uk) | |
Cultural and community facilities: number and area per resident—Cultural Infrastructure Map [2019] (https://data.london.gov.uk/dataset/cultural-infrastructure-map) | |
Public transport accessibility level (PTAL): PTAL levels—Transport for London [2015] (https://data.london.gov.uk/dataset/public-transport-accessibility-levels) | |
Land use: number of categories and dominant land use—UKLand [2020], EDINA Geomni Digimap Service (https://digimap.edina.ac.uk) | |
Building age: dominant building age—Dwelling Ages and Prices [2020] (https://data.cdrc.ac.uk/dataset/dwelling-ages-and-prices) | |
Green spaces: total green, % of green space, % of private green, and green density—public and private green space—same data source as mentioned on sports and play facilities (above) | |
Number and density of street trees: Local Authority Maintained Trees [2014–2015] (https://data.london.gov.uk/dataset/local-authority-maintained-trees#:~:text=This) | |
Air pollution: air quality levels (PM2.5) and affected area—London Atmospheric Emissions Inventory (LAEI) 2016—London Datastore | |
Noise: noise pollution levels (LAeq road/rail 16) and affected area—Noise Pollution in London [2012] (https://data.london.gov.uk/dataset/noise-pollution-in-london) | |
Socio-economic factors | Population: population density and net density—Population estimates [2019]—ONS Nomis (https://www.nomisweb.co.uk/) |
Jobs: workplace density—ONS Nomis Business Register and Employment Survey [2019] (https://www.nomisweb.co.uk/) | |
Overcrowding: household overcrowding—the proportion of all households in a defined area which are judged to have insufficient space to meet the household’s needs—same source as the Index of multiple deprivation (below) | |
Index of Multiple Deprivation (score): index created to identify the most deprived areas in England—Gov UK [2019] (https://www.gov.uk/government/statistics/english-indices-of-deprivation-2019) | |
Crime: total crime offences (year/1,000 residents)—London Metropolitan Police data [2019] (https://data.police.uk/) | |
Tenure type: private rental—ONS Households by tenure [2011] (https://www.nomisweb.co.uk/) | |
Car density: cars/1,000 residents—ONS Car or van availability [2011] (https://www.nomisweb.co.uk/) |
IoUH, Impact on Urban Health; MSOA, Middle-Layer Super Output Area; GIS, geographic information system; TFL, Transport for London; GP, general practitioner; NHS, National Health Service; PM, particulate matter; LAeq, average equivalent sound pressure level; ONS, Office for National Statistics.
A combination of different data sources within the UK, London and at the borough level, was used to compile a comparative and multi-dimensional analytical framework, which was in turn applied to each of the twelve neighbourhoods. The result is a multi-layered series of ‘magnetic resonance imaging (MRI)’ scans of each neighbourhood—with around 300 individual GIS-generated maps—which constitute a comprehensive spatial comparison of 12 specific locations in Southwark and Lambeth. All data was collected from December 2020 to June 2021. In some cases, due to limitation on data availability, measurement years and methodologies used to calculate indicator values may vary between boroughs and neighbourhoods and were not always be directly comparable. As seen in Table 2 the main data sources include Ordnance Survey data; London Datastore, UK Government data, CDRC data, Nomisweb data, and Lambeth and Southwark data. Data was collected at the most updated date and smaller unit of analysis, mostly MSOA and Lower-Layer Super Output Area (LSOA) levels.
These metrics were applied at the local scale, focusing on exploring London neighbourhoods within Southwark and Lambeth. The study examined geographical, spatial, and health level divergences, specifically focusing on parameters such as female healthy life expectancy, childhood obesity (in year 6, 10–11 years old), and diabetes prevalence. This instigated discussions, such as: (I) are similar socio-economic factors within neighbourhoods related with similar health outcomes? (II) Is there a positive relation between the availability of green infrastructure and sports facilities in neighbourhoods and health levels? (III) Are there visible differences in health outcomes between urban and suburban neighbourhoods? And do these disparities seem to relate to specific spatial factors? Aiming to address these and more questions by generating spatial and visual evidence, emphasizing the necessity for further and statistically rigorous studies.
The research methodology was based on GIS maps, while photography and site visits were used as complementary tools of analysis and to verify anomalies within the data. Mapping was used to help in different ways: (I) provide a spatial context that aids in understanding how health disparities are distributed across different neighbourhoods, visualising clusters, hotspots, or spatial trends that may be difficult to discern in tabular data; (II) identify spatial patterns and trends in health outcomes and their connections to specific spatial factors, leading to new insights or help confirm existing hypotheses; (III) incorporate a variety of geographic insights into the analysis, such as proximity to healthcare facilities, accessibility to transport, and environmental factors like pollution and green spaces. The visual nature of maps helped convey the importance of the research more effectively. In summary, incorporating mapping as an analytical and visual method provided a comprehensive and alternative method that considered both quantitative and spatial aspects in the relation between urban neighbourhoods and health.
Results
The conceptual model above (Figure 3) is a simple illustration on how various factors interact to influence health outcomes. However, this paper, due to its nature and methodology, will only explore potential links between socio-economic and environmental factors to health outcomes. While acknowledging the complexity of the system (35-38) and the presence of other contributing factors, it’s important to note that the project’s objective, the sample size, and the type of spatial analysis employed results in a simplified model of the entire system.
This section compares in greater depth how variations in the measurements of the three specific health outcomes are related with the range of attributes included in spatial analysis of the 12 selected neighbourhoods. To better understand some of the differences between the neighbourhoods, a 3D perspective of these areas can be observed in Figure 4.
As part of the research outputs a comparative ‘spider’ or ‘radar’ chart was created to display data across several dimensions and using visual organization and colour to help the analysis (see Figure 5). As mentioned before, for a better comparison and analysis, the neighbourhoods were clustered in groups of four—each cluster presenting contrasting levels for one of the health indicators, i.e., four neighbourhoods for female healthy life expectancy, four for obesity in year 6 and four for diabetes prevalence. For a more direct comparison, within each of the clusters, all graphs were ranked in order of health performance, from areas with lower health levels to areas with better health performance.
These diagrams feature a smaller sample of indicators—only 16—to simplify the analysis, avoid overlaps, and maintain a more balanced set of measures across various dimensions. The final selection was made based on their relevance in spatial, social, and environmental terms, capturing the individual ‘qualities’ of each neighbourhood. These selected indicators are then presented alongside the measures of health outcomes.
The variables are organised in groups, with the health indicators identified in blue, followed by the socio-spatial context indicators—connectivity, % built-up land, average building heights, social housing states density, % private rented housing, population density, overcrowding, multiple deprivation, accessibility to sports facilities and cultural/community facilities; and the environmental indicators—% of green space, green density (amount of green space per person) and tree density—all in black. Each dimension’s range is normalized to one another, so that the length of a line from zero to one is the same for every dimension1.
This spider matrix allows a comparative reading between neighbourhoods with negative and positive health outcomes. This high-level review of the spider charts for each of the twelve neighbourhoods highlights some of the main differences and similarities found between their health, spatial and socio-economic indicators. The analysis of the spider matrix shows an inverse relationship between positive health outcomes and the surface area of the spider charts. The smaller the area of the spider charts, aside from a few peaks associated with the green space indicators, the better the health outcomes.
An important output from reading these spider charts, is the similarity between the three groups of four diagrams. Similar patterns of characteristics demonstrate a relationship with the three health outcomes in comparable ways. For instance, similar socio-spatial characteristics tend to emerge in less healthy areas when compared with healthier areas. For the less healthy areas these include factors, or combination of factors, as deprivation and overcrowding, together with the presence of social housing estates, low density of green or dominance of private green areas, and lack of social and sports facilities. As an example, socio-economic indicators such as overcrowding and deprivation are strongly associated with neighbourhoods such as Tulse Hill, Stockwell East and Peckham North West compared with Herne Hill, Peckham Rye Common and West Dulwich.
In terms of spatial and environmental indicators, a positive relation between the healthier outcomes and the presence of large green public spaces, sports and play facilities, and cultural and community services can be seen. Access to social and cultural facilities is also not equitably distributed between areas.
More than the presence of green space per se, it seems to be the combination between the percentage of green, its ownership (private or public green space) and residential densities, that has a stronger relation with health outcomes. Two of the three healthier areas have high levels of public green and low residential densities (see Herne Hill & Dulwich Park and Peckham Rye Common). While areas with higher residential areas and lower levels of green space, in addition to a significant percentage of private green space, present poorer health outcomes (see Tulse Hill and Stockwell East for example). The interpretation of the diagrams also show that tree density is not directly related to health outcomes, even though trees have an important impact on health, pollution, and street attractiveness (39-41).
It is also important to note that overcrowding does not always relate to densely built. Many of the overcrowded areas have taller buildings and higher levels of open space (see Tulse Hill and Burgess Park). Most of the areas with higher levels of housing estates also emerge to be overcrowded, and in turn have high deprivation levels. In contrast, healthier areas tend to have low residential densities, lower percentages of built-up areas and less social housing—and with higher levels of private rental or house ownership.
Low residential areas with presence of large green spaces emerge connected to less road connectivity with impact on walkability and higher levels of car ownership. However, these areas with low connectivity are also areas with better health levels (see Herne Hill & Dulwich Park and Peckham Rye Common for example).
Discussion
This section summarises some of the key findings of the research, while highlighting some patterns and relationships between the selected socio-economic, spatial, and environmental indicators, and how these interact and impact on health-related issues. These patterns and relationships are presented in the summary below:
- Neighbourhoods with better health outcomes tend to have more green space and recreational facilities, sometimes supplemented by private green spaces. They present lower population and building density, and less deprivation. Conversely, areas with worse health outcomes are often overcrowded, deprived, and have limited public green spaces, much of which is private. Large parks compensate for the lack of formal play and sports facilities.
- There is a clear relationship between health levels, overcrowding, and deprivation, with more deprived areas having worse health outcomes. However, not all densely populated areas are overcrowded or deprived. Overcrowding also seems to be associated with higher crime levels, and areas with more crimes are often more deprived and have lower health levels.
- Tenure is important to consider. Private rentals are also associated with health outcomes, with owner-occupied areas generally showing better health outcomes. On the other hand, areas with higher concentrations of housing estates are linked to overcrowding and deprivation, which can influence health outcomes. Demonstrating that overcrowding and health is relevant, when discussing social housing.
- Geographical centrality plays a mixed role when exploring health. (I) Central areas have higher access to public and civic spaces, cultural and community facilities. These central areas also feature mixed land use and a higher presence of jobs, leading to a “24-hour population” profile which could seem to relate to better health outcomes. However, some of these areas have contrasting health levels (see for example the levels of healthy female life expectancy in Bermondsey East and North); (II) central areas have better transportation infrastructure, but also suffer from higher levels of air and noise pollution due to increased traffic which also contributes to this mixed role. At the same time, central areas have better connectivity and walkability which often relates to a healthier lifestyle. In comparison, suburban areas with larger green spaces often emerge with lower connectivity and walkability levels and higher car densities due to less public transport accessibility but not necessarily worse in terms of health outcomes (see for example West Dulwich).
Even though this research attempts to relate urban characteristics and health, it is not an in-depth analytical assessment that allows to correlate both factors. Important to highlight that there is no intention to find correlations or causation between the urban setting and the health levels of an area as this investigation was not conducted in a controlled environment, or through a combination of experimental evidence and a comprehensive and in-depth statistical analysis.
This study is an overview of selected data and a descriptive exploration of the current state the neighbourhoods’ urban characteristics. The use of GIS analysis was important to shed light on relationships between health outcomes and socio-environmental factors in a comparative way, particularly when analysing the physical and built-up environment.
Some important patterns can however be inferred from this comparative overview. The documentation of those patterns and how they relate to contextual and environmental characteristics might help draw attention for strategic measures that might be needed to prevent or mitigate socio-spatial inequalities (13).
This research was mostly developed during COVID-19 lockdown and with limitations to any fieldwork or access to the areas. Most data were collected through desktop research or through access to open access databases, with some methodological limitations and comparative limitations in a few cases. Nevertheless, this investigation and its methodology will hopefully provide a solid resource for academics, and policy makers, while informing future research opportunities. These could be an expansion of the selected set of indicators to other health issues, or an exploration of factors related to individual behaviour—as food and drinking consumption, lifestyles, routines, etc. In the future, processes of engagement with residents and key actors could also provide more in-depth results on how space conditions might inform health outcomes. Also, the analysis of future scenarios that consider, at the same time, the current changes in these areas and the strategic policies proposed for London, could be an opportunity to think ahead and try to understand potential impacts on local urban health.
Conclusions
In conclusion, this study uses spatial mapping and visual analysis to provide valuable insights into the intricate relationship between neighbourhoods and health, underscoring the multifaceted nature of this dynamic. It reveals several key relationships among factors influencing health outcomes within these neighbourhoods. Notably, addressing socio-economic disparities, and the need to employ a more comprehensive set of indicators in urban planning and management. The study identifies negative associations between health and socio-economic factors like overcrowding and deprivation, as well as the significance of accessible public green spaces for better health outcomes. Furthermore, it shows that the presence of community services, sports facilities, and play amenities seems to positively influence health, even in densely populated areas. It shows that in London, geographical location brings mixed results when relating to health and that tenure, in particular social housing metrics, are important to consider when exploring health.
This descriptive analysis, highlights the potential for holistic interventions, encompassing improvements in housing quality, support for active lifestyles, and enhanced environmental management, to ameliorate health outcomes and reduce health disparities. These findings underscore the importance of ongoing spatial research and targeted actions to foster healthier communities and mitigate health inequalities.
Acknowledgments
The authors are indebted to Rob Parker, Claire Benard, Alessandra Denotti and Anna Tarkington (IoUH) for their support and contribution to this work, and to Bethany Mickleburgh and Astrid Walker-Stewart (LSE Cities) for their invaluable work.
Funding: This work was supported by
Footnote
In the case of life expectancy, and to increase comparability with the other two health indicators, ‘healthy female life expectancy’ was replaced by the ‘difference in female life expectancy’, representing the difference between the highest value of all areas, and the value of each neighbourhood.
Provenance and Peer Review: This article was commissioned by the Guest Editors (Michael K. Gusmano & Victor G. Rodwin) for the series “Health Systems and Health in World Cities: Challenges for the Future” published in Journal of Hospital Management and Health Policy. The article has undergone external peer review.
Peer Review File: Available at https://jhmhp.amegroups.com/article/view/10.21037/jhmhp-23-138/prf
Conflicts of Interest: Both authors have completed the ICMJE uniform disclosure form (available at https://jhmhp.amegroups.com/article/view/10.21037/jhmhp-23-138/coif). The series “Health Systems and Health in World Cities: Challenges for the Future” was commissioned by the editorial office without any funding or sponsorship. A.G. and R.B. received support from Impact on Urban Health. The authors have no other conflicts of interest to declare.
Ethical Statement: The authors are accountable for all aspects of the work in ensuring that questions related to the accuracy or integrity of any part of the work are appropriately investigated and resolved. Publication of this paper adheres to the LSE Research Ethics Policy and Procedures, and it only uses publicly available and anonymized data.
Open Access Statement: This is an Open Access article distributed in accordance with the Creative Commons Attribution-NonCommercial-NoDerivs 4.0 International License (CC BY-NC-ND 4.0), which permits the non-commercial replication and distribution of the article with the strict proviso that no changes or edits are made and the original work is properly cited (including links to both the formal publication through the relevant DOI and the license). See: https://creativecommons.org/licenses/by-nc-nd/4.0/.
References
- The British Academy COVID-19 and Society. The COVID Decade. Understanding the long-term societal impacts of COVID-19 [Internet]. London, UK: The British Academy; 2021:172. [cited 2023 Sep 16]. Available online: https://www.thebritishacademy.ac.uk/documents/3238/COVID-decade-understanding-long-term-societal-impacts-COVID-19.pdf
- Mouratidis K. How COVID-19 reshaped quality of life in cities: A synthesis and implications for urban planning. Land use policy 2021;111:105772. [Crossref] [PubMed]
- UN-Habitat. Cities and Pandemics: Towards a more just, green and healthy future | UN-Habitat [Internet]. Nairobi, Kenya: UN-Habitat; 2021:194. [cited 2023 Dec 21] Available online: https://unhabitat.org/cities-and-pandemics-towards-a-more-just-green-and-healthy-future-0
- Neuman M, Chelleri L, Schuetze T. Post-Pandemic Urbanism: Criteria for a New Normal. Sustainability 2021;13:10600. [Crossref]
- Roxberg Å, Tryselius K, Gren M, et al. Space and place for health and care. Int J Qual Stud Health Well-being 2020;15:1750263. [Crossref] [PubMed]
- Diez Roux AV. Neighborhoods and Health: What Do We Know? What Should We Do? Am J Public Health 2016;106:430-1. [Crossref] [PubMed]
- Mohnen SM, Schneider S, Droomers M. Neighborhood characteristics as determinants of healthcare utilization - a theoretical model. Health Econ Rev 2019;9:7. [Crossref] [PubMed]
- Hilary G. Understanding Health Inequalities. McGraw-Hill Education (UK); 2009:241.
- Gatrell AC, Popay J, Thomas C. Mapping the determinants of health inequalities in social space: can Bourdieu help us? Health Place 2004;10:245-57. [Crossref] [PubMed]
- Curtis S, Rees Jones I. Is There a Place for Geography in the Analysis of Health Inequality? Sociology of Health & Illness 1998;20:645-72. [Crossref]
- Gatrell AC, Elliott SJ. Geographies of Health: An Introduction. John Wiley & Sons; 2014:434.
- Shaw M, Dorling D, Mitchell R. Health, Place, and Society. Pearson Education; 2002:236.
- Frumkin H. Health, equity, and the built environment. Environ Health Perspect 2005;113:A290-1. [Crossref] [PubMed]
- Koehler K, Latshaw M, Matte T, et al. Building Healthy Community Environments: A Public Health Approach. Public Health Rep 2018;133:35S-43S. [Crossref] [PubMed]
- Diez-Roux AV, Link BG, Northridge ME. A multilevel analysis of income inequality and cardiovascular disease risk factors. Soc Sci Med 2000;50:673-87. [Crossref] [PubMed]
- Duncan C, Jones K, Moon G. Context, composition and heterogeneity: using multilevel models in health research. Soc Sci Med 1998;46:97-117. [Crossref] [PubMed]
- Ribeiro AI, Gullón P, Murray ET. Neighbourhood Influences on Population Health: Time to Unpack the Black Box. Public Health Rev 2022;43:1605053. [Crossref] [PubMed]
- Remme RP, Frumkin H, Guerry AD, et al. An ecosystem service perspective on urban nature, physical activity, and health. Proc Natl Acad Sci U S A 2021;118:e2018472118. [Crossref] [PubMed]
- Future of London. Healthy Neighbourhoods [Internet]. Future of London. 2022 [cited 2023 Sep 16]. Available online: https://www.futureoflondon.org.uk/knowledge/healthy-neighbourhoods/
- Lowe M, Foster S. Neighbourhood characteristics and health: advancing research and practice. Lancet Public Health 2021;6:e351-2. [Crossref] [PubMed]
- Kivimäki M, Batty GD, Pentti J, et al. Modifications to residential neighbourhood characteristics and risk of 79 common health conditions: a prospective cohort study. Lancet Public Health 2021;6:e396-407. [Crossref] [PubMed]
- Ribeiro AI. Public health: why study neighborhoods? Porto Biomed J 2018;3:e16. [Crossref] [PubMed]
- Grigoroglou C. A spatial analysis of neighbourhood contextual factors and their association with mental and physical health outcomes for the Primary Care Population of England. [Internet]. 2020 [cited 2023 Sep 18]. Available online: chrome-extension://efaidnbmnnnibpcajpcglclefindmkaj/https://research.manchester.ac.uk/files/177285593/FULL_TEXT.PDF
- Bissonnette L, Wilson K, Bell S, et al. Neighbourhoods and potential access to health care: the role of spatial and aspatial factors. Health Place 2012;18:841-53. [Crossref] [PubMed]
- Root ED. Moving Neighborhoods and Health Research Forward: Using Geographic Methods to Examine the Role of Spatial Scale in Neighborhood Effects on Health. Ann Assoc Am Geogr 2012;102:986-95. [Crossref] [PubMed]
- Macintyre S, Maciver S, Sooman A. Area, Class and Health: Should we be Focusing on Places or People? Journal of Social Policy 1993;22:213-34. [Crossref]
- Macintyre S, Ellaway A, Cummins S. Place effects on health: how can we conceptualise, operationalise and measure them? Soc Sci Med 2002;55:125-39. [Crossref] [PubMed]
- Park YM, Kwan MP. Multi-Contextual Segregation and Environmental Justice Research: Toward Fine-Scale Spatiotemporal Approaches. Int J Environ Res Public Health 2017;14:1205. [Crossref] [PubMed]
- Fayet Y, Praud D, Fervers B, et al. Beyond the map: evidencing the spatial dimension of health inequalities. Int J Health Geogr 2020;19:46. [Crossref] [PubMed]
- Suel E, Polak JW, Bennett JE, et al. Measuring social, environmental and health inequalities using deep learning and street imagery. Sci Rep 2019;9:6229. [Crossref] [PubMed]
- LSE Cities. Urban Age [Internet]. 2021 [cited 2024 Jan 9]. Available online: https://urbanage.lsecities.net/
- Heeckt C, Gomes A, Ney D, et al. Towards Urban Growth Analytics for Yangon [Internet]. London, UK: LSE Cities; 2017. [cited 2024 Jan 9]. Available online: https://www.lse.ac.uk/Cities/publications/research-reports/Towards-Urban-Growth-Analytics-for-Yangon.aspx
- Galster G. On the Nature of Neighbourhood. Urban Studies 2001;38:2111-24. [Crossref]
- Lebel A, Pampalon R, Villeneuve PY. A multi-perspective approach for defining neighbourhood units in the context of a study on health inequalities in the Quebec City region. Int J Health Geogr 2007;6:27. [Crossref] [PubMed]
- Kent County Council. Planning influences the wider determinants of health [Internet]. Health, Planning and Sustainability Toolkit. [cited 2024 Jan 10]. Available online: https://healthsustainabilityplanning.co.uk/health/why-engage-with-planning/planning-influences-wider-determinants-health/
- Pineo H. Towards healthy urbanism: inclusive, equitable and sustainable (THRIVES) – an urban design and planning framework from theory to praxis. Cities & Health 2022;6:974-92. [Crossref]
- Public Health England. Spatial planning for health: evidence review [Internet]. UK: Public Health England; 2017:69 [cited 2024 Jan 10] (PHE publications). Available online: https://www.gov.uk/government/publications/spatial-planning-for-health-evidence-review
- World Health Organisation. A Conceptual Framework for Action on the Social Determinants of Health [Internet]. Geneva: WHO; 2010:76. [cited 2024 Jan 10]. Available online: https://www.who.int/publications/i/item/9789241500852
- Mullaney J, Lucke T, Trueman SJ. A review of benefits and challenges in growing street trees in paved urban environments. Landscape and Urban Planning 2015;134:157-66. [Crossref]
- Douglas I, Goode D, Houck MC, et al. Handbook of Urban Ecology. Routledge; 2010:689.
- Wolf KL, Lam ST, McKeen JK, et al. Urban Trees and Human Health: A Scoping Review. Int J Environ Res Public Health 2020;17:4371. [Crossref] [PubMed]
Cite this article as: Gomes A, Burdett R. Spatial and visual comparison analysis of health disparities in London neighbourhoods: the case of Southwark and Lambeth. J Hosp Manag Health Policy 2024;8:5.